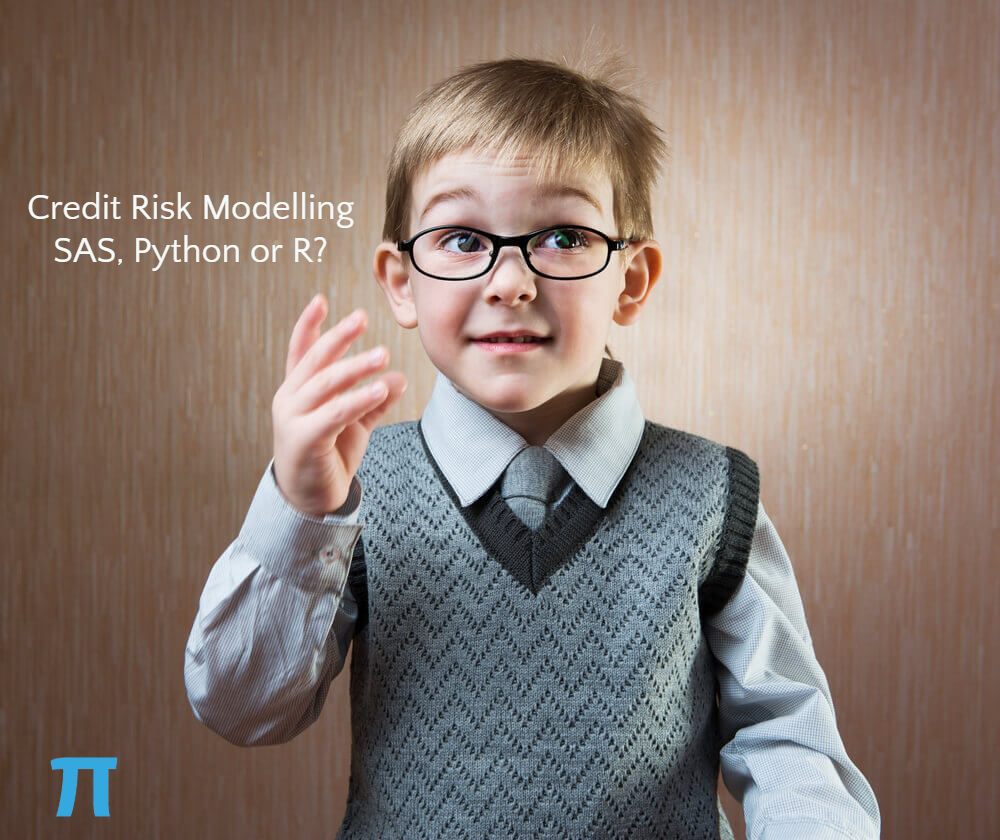
Sep
Credit Risk Modelling – SAS, Python or R?
Technology is continually evolving. It is futile to ever expect anything to stay the same for too long where tech is concerned. Some ecosystems evolve fast, some move at a more mundane pace. For a very long time, SAS has been the language of choice for Credit Risk modelling, a package that has been around since 1979. I even recall using it in my Statistics module in my degree 20 years ago! Fast forward to the early 90s, Python and R came along and as of now, they are the primary challengers to SAS within the Credit Risk modelling arena. R is now used in statistics modules in universities in place of SAS and importantly, both Python and R are free and open source to boot!
Python seems to be able to do everything, whether you want to use it for low touch scripts, powering websites all the way to full blown/large scale programming and now even (Credit) Risk modelling. It really is an all-round, general purpose language that has gained massive traction in the financial services world in particular. R is a language and environment that was designed specifically for statistical computing and graphics, a GNU project, similar to the S language. Moving SAS aside for one moment, when it comes to Python and R with respect to modelling, it is a case of what one would you prefer – that is, do you want apples or pears? They are both considered very close contenders by the Quant and Analytics community and It can make for a difficult decision choosing one over the other when applying them to Credit Risk modelling. Many factors need to be taken into account, mainly centered around what it is you want to achieve, as well as the pre-processing and graphical capabilities. There is no pre-determined criteria for choosing one over the other, it needs to really be taken on a case by case basis.
One of the key things to consider however, is the simplicity of Python compared to the very steep learning curve that comes with R. Due to this extra complexity with R, those that do know R to a very high standard have acquired a certain kudos in what is a very niche space resulting in higher employability rates where it is needed. Due to Python and R being “newer” and cheaper than SAS, it naturally makes sense that starts ups and small to mid-size firms will adopt one of the two of them. SAS however, has a very strong legacy foothold within larger corporations with big budgets and once it is implemented, it is usually maintained resulting in a buoyant marketplace for SAS orientated Credit Risk Quants in the predominantly larger institutions.
Credit Risk modelling using R, Python and other analytics friendly programming languages has greatly improved the ease and accuracy of Credit Risk modelling. Credit Risk modelling is still extremely niche and offers great opportunities for those who have a strong grasp of analytics combined with the world of finance. In this respect, SAS still holds the largest market share and from what we are currently seeing at Quantribute, if you are a Credit Risk Quant, you should look at knowing either SAS and Python or R and Python as a combination (or all three if possible!).
Having said this, we definitely see Python becoming more and more prevalent with its powerful packages/libraries (numpy, scipy, scikit-learn, matplotlib) and functions for almost any statistical operation/model building you may want to do. In particular, since the introduction of pandas, it has become very strong in operations on structured data. It makes sense to combine Python with the market leader in commercial analytics – SAS, or it’s open source challenger – R to give yourself as much optionality when it comes to employability across different sizes and shapes of organisations.
If you are a Credit Risk Quant interested in the UK and European quant jobs market and what opportunities are out there, get in touch for a friendly and professional chat.
– Saj Samiullah (Director, Quantribute)
Sorry, the comment form is closed at this time.